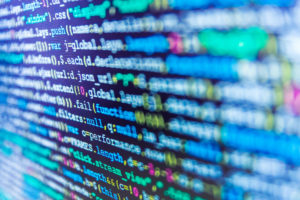
Data scientists spend about 45% of their time on data preparation tasks, including loading and cleaning data, according to a survey of data scientists conducted by Anaconda. The company also analyzed the gap between what data scientists learn as students, and what the enterprises demand.
Data cleansing – fixing or discarding anomalous or wrong numbers and otherwise ensuring the data is an accurate representation of the phenomenon it is meant to measure — accounts for more than a quarter of average day for data scientists, followed by 19% for data loading (the “L” in ETL), according to Anaconda’s annual survey.
Data visualization tasks occupied for about 21% of their time, while model selection, model training and scoring, and model deployment each consume 11% to 12% of the day, the survey found.
“We were disappointed, if not surprised, to see that data wrangling still takes the lion’s share of time in a typical data professional’s day,” Anaconda wrote in its report, “2020 State of Data Science: Moving From Hype Toward Maturity.” “Data preparation and cleansing takes valuable time away from real data science work and has a negative impact on overall job satisfaction.”
It could be worse. In some surveys in the past, data prep tasks have occupied upwards of 70% to 80% of a data scientist’s time. That is why so many people have questioned the wisdom of asking highly skilled and highly paid data scientists to do the equivalent of digital janitorial work.
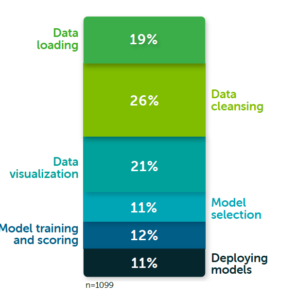
How data scientists spend their time (Image courtesy Anaconda “2020 State of Data Science: Moving From Hype Toward Maturity.”)
So, the sticky situation around asking data scientists to spend the bulk of their time preparing data for analysis continues. “This efficiency gap presents an opportunity for the industry to work on solutions to this problem, as one has yet to emerge,” Anaconda laments.
The 2020 State of Data Science is based on online surveys of nearly 2,400 people from more than 100 countries (not all of whom are data scientists themselves, although they work in the field). In addition to asking about common data science tasks, Anaconda inquired into the languages data scientists use, their favored toolkits, as well as identifying barriers to deployment of machine learning models and adoption of open source technology by other members of the data science team: developers, administrators, and line of business managers.
To no one’s surprise, Python dominated the language question. According to Anaconda’s survey, 47% of data scientists say they “always” use Python, while another 28% say they use it “frequently.” By comparison, only 10% of respondents say they “always” use R, which was the second most-used language in the survey. JavaScript, Java, C, C++, and C# were all in the mix, but Python simply dwarfed (or suffocated?) them in usage.
When it came to data science, it should come as no surprise that Anaconda’s own data science platform—which combines many of the most commonly used tools in the Python and R ecosystems into one easy-to-use bundle–was cited as the most-used toolkit (the sample of users Anaconda used for the survey may have had something to do with that). Interestingly, Anaconda says 44% of its users also use RStudio, which develops a suite of open source tools for R (and Python too).
To read the full article, visit https://www.datanami.com/2020/07/06/data-prep-still-dominates-data-scientists-time-survey-finds/